One step further towards day-ahead solar variability prediction
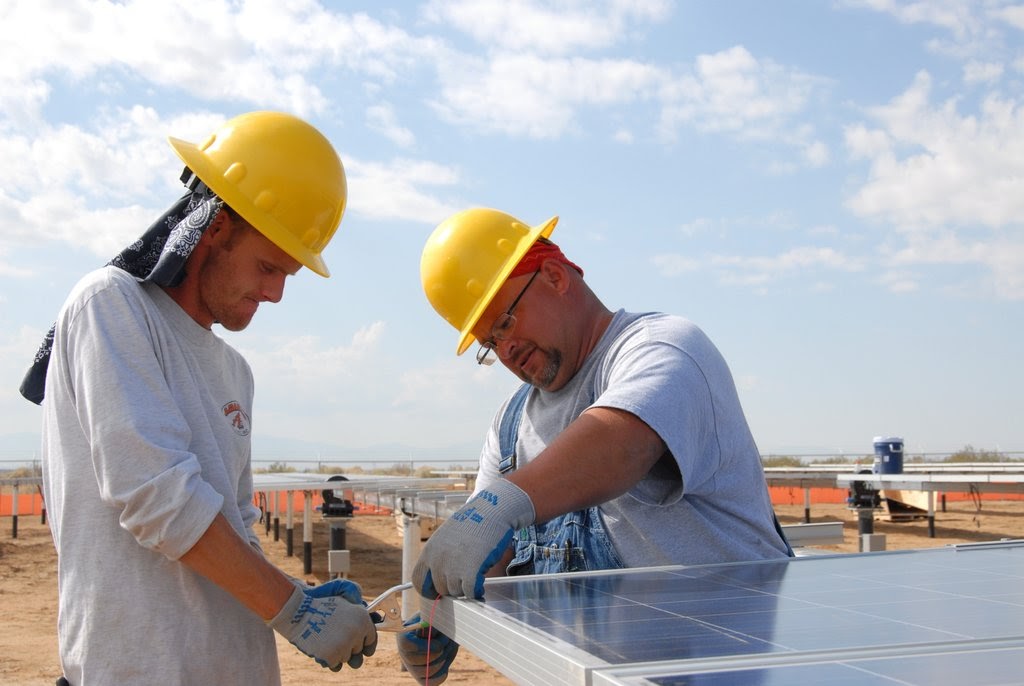
New research led by GML/CIRES scientist Laura Riihimaki was published in Solar Energy on July 23, funded by NOAA Atmospheric Science for Renewable Energy Development and the Department of Energy Solar Energy Technologies Office.
The study characterized the relationship of solar irradiance variability with cloud properties and quantified that relationship using machine learning models. Observations were taken from the Department of Energy Atmospheric Radiation Measurement site in Oklahoma.
Seasonal analysis suggests the relationship is relatively weather regime and location independent. Machine learning models developed to quantify the solar irradiance variability-cloud relationship showed the best performance with an R2 of 0.42.
The data and model are being used to create a numerical weather prediction model for day-ahead solar variability prediction. This is a first step towards developing a new tool to improve grid operation, planning, and resilience for renewable power generation.
The ability to predict solar irradiance variability might help mitigate some effects of resource variability caused by high solar power penetration into the electricity grid during renewable power generation.
Temporal variability can also be reduced with spatial averaging so that segments of the solar industry that depend on the variability over a wider geographic region reduce some of the need to predict high temporal resolution variability.